Statistical analysis of DIA data


Overview
Questions:Objectives:
How to perform statistical analysis on DIA mass spectrometry data?
How to detect and quantify differentially abundant proteins in a HEK-Ecoli Benchmark DIA datatset?
Requirements:
Statistical analysis of a HEK-Ecoli Benchmark DIA dataset
Understanding statistical approaches in proteomic analysis
Using MSstats to find significantly dysregulated proteins in a Spike-in dataset
- Introduction to Galaxy Analyses
- Proteomics
- Library Generation for DIA Analysis: tutorial hands-on
- DIA Analysis using OpenSwathWorkflow: tutorial hands-on
Time estimation: 1 hourLevel: Intermediate IntermediateSupporting Materials:Last modification: Aug 6, 2021
Introduction
This training covers the statistical analysis of data independent acquisition (DIA) mass spectrometry (MS) data, after successfull identification and quantification of peptides and proteins. We therefore recommend to first go through the DIA library generation tutorial as well as the DIA analysis tutorial, which teach the principles and characteristics of DIA data analysis.
Modern mass spectrometry approaches enables the identification and quantification of thousands of proteins and tens of thousands of peptides in single measurements. This provides immense potential to in-depth explorative analysis of a variety of biological samples. However, often the number of available samples is limited leading to large proteomic datasets with only a few numbers of replicates or samples per condition. Thus, the statistical analysis remains challenging in such in-depth proteomic studies.
Here we will use MSstats, which enables the statistical analysis and processing of proteomic data (Choi et al. 2014).
Agenda
In this tutorial, we will cover:
Get data
hands_on Hands-on: Data upload
Create a new history for this tutorial and give it a meaningful name
Tip: Creating a new history
Click the new-history icon at the top of the history panel.
If the new-history is missing:
- Click on the galaxy-gear icon (History options) on the top of the history panel
- Select the option Create New from the menu
- Import the DIA analysis results, the sample annotation and the comparison matrix from Zenodo
https://zenodo.org/record/4307758/files/PyProphet_export.tabular https://zenodo.org/record/4307758/files/Sample_annot_MSstats.txt https://zenodo.org/record/4307758/files/Comp_matrix_HEK_Ecoli.txt https://zenodo.org/record/4307758/files/PyProphet_msstats_input.tabular
Tip: Importing via links
- Copy the link location
Open the Galaxy Upload Manager (galaxy-upload on the top-right of the tool panel)
- Select Paste/Fetch Data
Paste the link into the text field
Press Start
- Close the window
Once the files are green, rename the sample annotation file in ‘Sample_annot_MSstats’, the comparison matrix file in ‘Comp_matrix_HEK_Ecoli’ and the two DIA analysis results files in ‘PyProphet_export’ and ‘PyProphet_msstats_input’
Tip: Renaming a dataset
- Click on the galaxy-pencil pencil icon for the dataset to edit its attributes
- In the central panel, change the Name field
- Click the Save button
Statistical analysis with MSstats
hands_on Hands-on: Performing statistical analysis using MSstats and the tabular output from PyProphet export
- MSstats Tool: toolshed.g2.bx.psu.edu/repos/galaxyp/msstats/msstats/3.20.1.0 with the following parameters:
- “input source”:
OpenSWATH
- param-file “OpenSWATH_input”:
PyProphet_export
- param-file “OpenSWATH_annotation”:
Sample_annot_MSstats
- “Compare Groups”:
Yes
- param-file “Comparison Matrix”:
Comp_matrix_HEK_Ecoli
comment Comment: data Process and group comparison
During the data process step the peptide intensities are normalized and protein inference is performed. Using a predefined comparison matrix multiple comparisons can be performed.
question Questions
- How many lines does the PyPyprophet_export.tabular file have? How many lines does the ProcessedData have and do you notice any differences in their structure or format?
- How many proteins were used for the Group comparison? (see ComparisonResult)
solution Solution
- The PyPyprophet_export.tabular has appr. 230.000 lines whereas the ProcessedData has over 1 mio lines and is in the so called long format. Here every individual transition (single m/z value) is reported per row.
- The ComparisonResult has 5022 lines, meaning over 5000 Proteins were compared between the two different Spike-in conditions.
tip Tip: Continue with results from Zenodo
In case the MSstats run is not yet finished, the results can be downloaded from Zenodo to be able to continue the tutorial
- Import the files from Zenodo
https://zenodo.org/record/4307758/files/MSstats_ComparisonResult_export_tabular.tsv
Detailed investigation of Ecoli identifications and quantifications
hands_on Hands-on: Investigating Ecoli proteins in the MSstats comparison results
- Select Tool: Grep1 with the following parameters:
- param-file “Select lines from”:
MSstats_ComparisonResult_export_tabular
(output of MSstats tool)- “the pattern”:
(ECOLI)|(log2FC)
- Filter Tool: Filter1 with the following parameters:
- param-file “Filter”:
Select_Ecoli
(output of Select tool)- “With following condition”:
c7!='NA'
- “Number of header lines to skip”:
1
- Histogram Tool: toolshed.g2.bx.psu.edu/repos/devteam/histogram/histogram_rpy/1.0.4 with the following parameters:
- param-file “Dataset”:
Filter_Ecoli
(output of the previous Filter tool)- “Numerical column for x axis”:
Column: 3
- “Number of breaks (bars)”:
25
- “Plot title”:
Distribution of Ecoli Protein log FC values
- “Label for x axis”:
log2 Fold Change
comment Comment: Extracting Ecoli information
First we only select rows containing specific terms such as “Ecoli” from the complete ComparisonResults file. Afterwards, the table is filtered to containg only proteins with valid statistical information (e.g. p-value). Using the log2 Fold change values from all remaining Ecoli proteins we can observe the distribution of log2FC values from the comparison of the two Spike-in conditions.
question Questions
- How many Ecoli proteins were identified and for how many was the p-value for the comparison of the two Spike-in conditions computed?
- How does the distribution of the log2FC values look like? Which Spike-in contained higher amounts of Ecoli and is it possible to see how much more Ecoli was spiked-in?
solution Solution
- In total, over 800 Ecoli proteins were identified from which 500 have a p-value for the comparison of the two Spike-in conditions.
- We can see a gaussian distribution of the log2FC values around a positive value of 3. Since we compared Spike_in_2 / Spike_in_1 we can directly see that Spike_in_2 contained higher amounts of Ecoli. Furthermore, since the apex of the distribution is around 3 and we compared log2 intensities, we could estimate that Spike_in_2 contained approx. 8-times more Ecoli than Spike_in_1.
Statistical analysis with MSstats
hands_on Hands-on: Performing statistical analysis using MSstats and the msstats_input from PyProphet export
- MSstats Tool: toolshed.g2.bx.psu.edu/repos/galaxyp/msstats/msstats/3.20.1.0 with the following parameters:
- “input source”:
MStats 10 column format
- param-file “MSstats 10-column input”:
PyProphet_msstats_input
- “Compare Groups”:
Yes
comment Comment: MSstats input format
For the statistical analysis using MSstats the input must be in the long format, containing all relevant information in 10 predefined columns. The conversion of the PyProphet export output can either be done using MSstats (as we did above), or during the Pyprophet export step by using another R package called swath2stats (Blattmann et al. 2016). Prior to the conversion the data can be processed and filtered using the swath2stats functionalities.
question Questions
- How many lines does the
PyProphet_msstats_input.tabular
file have? How many lines does the ProcessedData have and do you notice any differences in their structure or format?- How many proteins were used for the Group comparison? And do you already see a difference to the first MSstats step?
solution Solution
- The
PyProphet_msstats_input.tabular
has over 870.000 lines and the ProcessedData has over 1 mio lines. Here both files are in the long format, in which every transition is reported per row.- Here the ComparisonResult has only 3871 lines, meaning that almonst 1200 fewer proteins were used in the comparison of the two spike-in conditions.
tip Tip: Continue with results from Zenodo
In case the MSstats run is not yet finished, the results can be downloaded from Zenodo to be able to continue the tutorial
- Import the files from Zenodo
https://zenodo.org/record/4307758/files/MSstats_ComparisonResult_msstats_input.tsv
Detailed investigation of Ecoli identifications and quantifications
hands_on Hands-on: Investigating Ecoli proteins in the MSstats comparison results
- Select Tool: Grep1 with the following parameters:
- param-file “Select lines from”:
MSstats_ComparisonResult_msstats_input
(output of the second MSstats tool)- “the pattern”:
(ECOLI)|(log2FC)
- Filter Tool: Filter1 with the following parameters:
- param-file “Filter”:
Select_Ecoli
(output of the second Select tool)- “With following condition”:
c7!='NA'
- “Number of header lines to skip”:
1
- Histogram Tool: toolshed.g2.bx.psu.edu/repos/devteam/histogram/histogram_rpy/1.0.4 with the following parameters:
- param-file “Dataset”:
Filter_Ecoli
(output of the previous Filter tool)- “Numerical column for x axis”:
Column: 3
- “Number of breaks (bars)”:
25
- “Plot title”:
Distribution of Ecoli Protein log FC values
- “Label for x axis”:
log2 Fold Change
question Questions
- How many Ecoli proteins were identified and for how many was the p-value for the comparison of the two Spike-in conditions computed? Are there any differences compared to the selected and filtered results from the previous MSstats step?
- How does the distribution of the log2FC values look like? Are there any differences compared to the selected and filtered results from the previous MSstats step?
solution Solution
- In total, over 600 Ecoli proteins were identified from which 500 have a p-value for the comparison of the two Spike-in conditions. Here we identify 200 Ecoli proteins less than before, however, the number of proteins for which a p-value was calculated differs only slightly.
- Generally, the two log2FC distribution look very similar, showing a gaussian distribution of the log2FC values around a positive value of 3. There seems to be a slight difference of the apex of the distribution, in the first MSstats analysis it seems to be higher than 3, whereas in the second MSstats analysis the apex seems to be lower than 3.
Conclusion
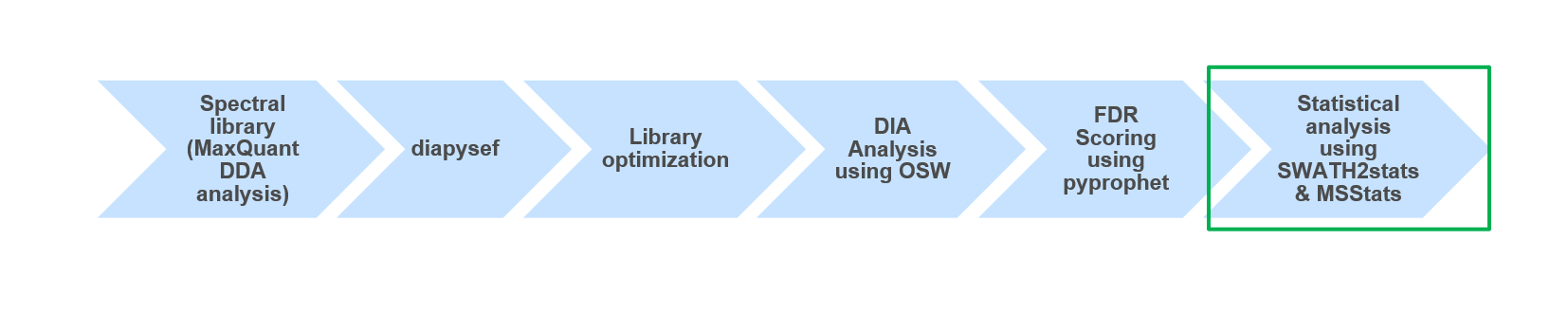
Using MSstats we were able to identify and quantify differentially regulated proteins between two Spike-in conditions in a HEK/Ecoli Benchmark DIA datatset. Furthermore, the preprocessing of the proteomic data prior to the statistical analysis can directly impact results. Thus, it might be beneficial to try various ways of intermediate data processing and statistical analysis to increase the sensitivity and specificity of the investigation.
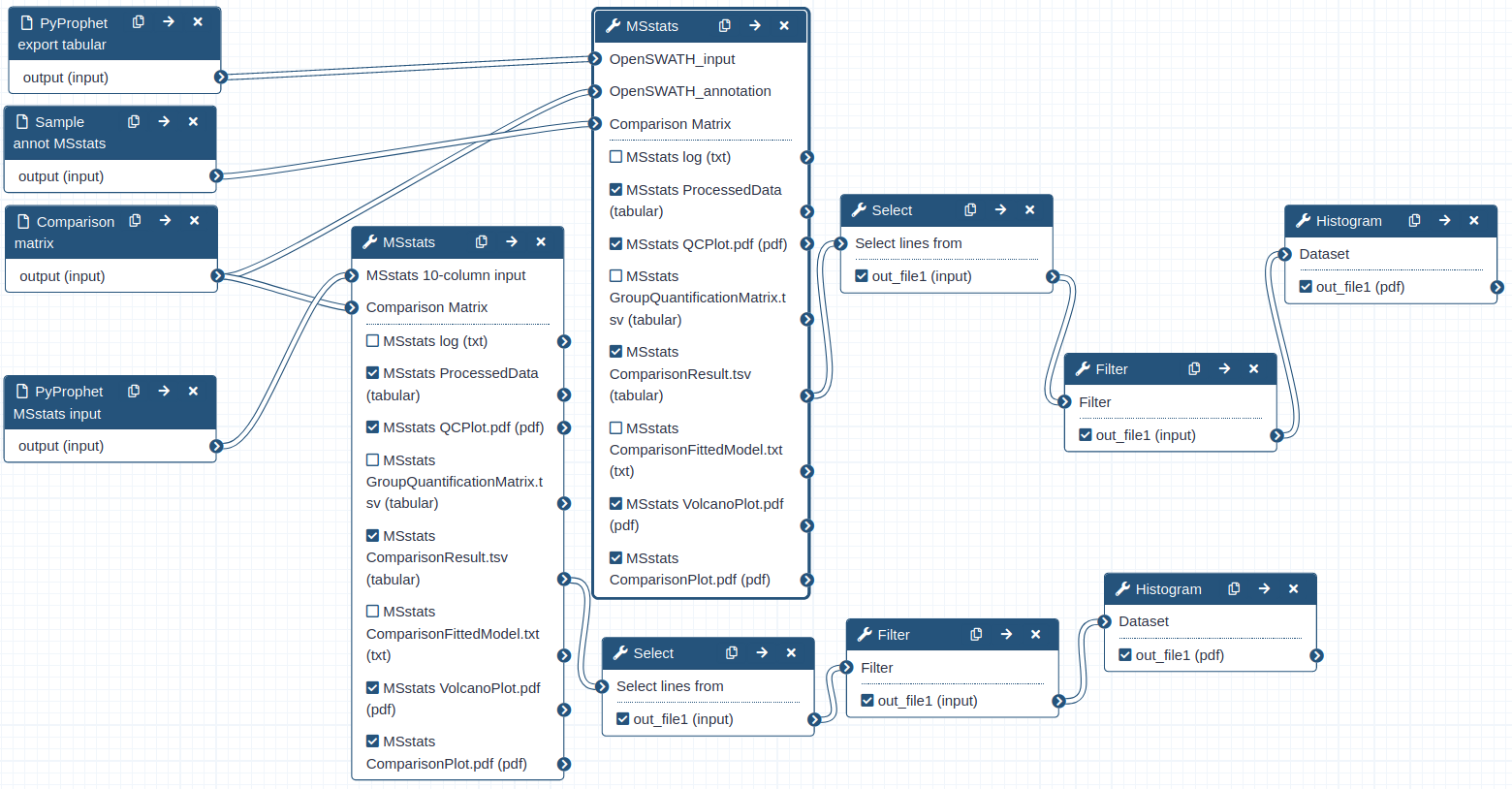
Key points
Statistical analyses are crucial for meaningful conclusion in proteomic studies
MSstats enables statistical analysis of data independent acquisition mass spectrometry data
Frequently Asked Questions
Have questions about this tutorial? Check out the tutorial FAQ page or the FAQ page for the Proteomics topic to see if your question is listed there. If not, please ask your question on the GTN Gitter Channel or the Galaxy Help ForumUseful literature
Further information, including links to documentation and original publications, regarding the tools, analysis techniques and the interpretation of results described in this tutorial can be found here.
References
- Choi, M., C.-Y. Chang, T. Clough, D. Broudy, T. Killeen et al., 2014 MSstats: an R package for statistical analysis of quantitative mass spectrometry-based proteomic experiments. Bioinformatics 30: 2524–2526. 10.1093/bioinformatics/btu305 https://academic.oup.com/bioinformatics/bioinformatics/article/2748156/MSstats:
- Blattmann, P., M. Heusel, and R. Aebersold, 2016 SWATH2stats: An R/bioconductor package to process and convert quantitative SWATH-MS proteomics data for downstream analysis tools. PLoS ONE 11: 1–7. 10.1371/journal.pone.0153160
Feedback
Did you use this material as an instructor? Feel free to give us feedback on how it went.
Did you use this material as a learner or student? Click the form below to leave feedback.
Citing this Tutorial
- Matthias Fahrner, Melanie Föll, 2021 Statistical analysis of DIA data (Galaxy Training Materials). https://training.galaxyproject.org/training-material/topics/proteomics/tutorials/DIA_Analysis_MSstats/tutorial.html Online; accessed TODAY
- Batut et al., 2018 Community-Driven Data Analysis Training for Biology Cell Systems 10.1016/j.cels.2018.05.012
details BibTeX
@misc{proteomics-DIA_Analysis_MSstats, author = "Matthias Fahrner and Melanie Föll", title = "Statistical analysis of DIA data (Galaxy Training Materials)", year = "2021", month = "08", day = "06" url = "\url{https://training.galaxyproject.org/training-material/topics/proteomics/tutorials/DIA_Analysis_MSstats/tutorial.html}", note = "[Online; accessed TODAY]" } @article{Batut_2018, doi = {10.1016/j.cels.2018.05.012}, url = {https://doi.org/10.1016%2Fj.cels.2018.05.012}, year = 2018, month = {jun}, publisher = {Elsevier {BV}}, volume = {6}, number = {6}, pages = {752--758.e1}, author = {B{\'{e}}r{\'{e}}nice Batut and Saskia Hiltemann and Andrea Bagnacani and Dannon Baker and Vivek Bhardwaj and Clemens Blank and Anthony Bretaudeau and Loraine Brillet-Gu{\'{e}}guen and Martin {\v{C}}ech and John Chilton and Dave Clements and Olivia Doppelt-Azeroual and Anika Erxleben and Mallory Ann Freeberg and Simon Gladman and Youri Hoogstrate and Hans-Rudolf Hotz and Torsten Houwaart and Pratik Jagtap and Delphine Larivi{\`{e}}re and Gildas Le Corguill{\'{e}} and Thomas Manke and Fabien Mareuil and Fidel Ram{\'{\i}}rez and Devon Ryan and Florian Christoph Sigloch and Nicola Soranzo and Joachim Wolff and Pavankumar Videm and Markus Wolfien and Aisanjiang Wubuli and Dilmurat Yusuf and James Taylor and Rolf Backofen and Anton Nekrutenko and Björn Grüning}, title = {Community-Driven Data Analysis Training for Biology}, journal = {Cell Systems} }